March 11, 2020
OCHIN researchers are analyzing the wealth of data available via the ADVANCE Collaborative to improve clinical screening and treatment for infectious diseases, like tuberculosis. In partnership with the Centers for Disease Control and Prevention (CDC), and the National Network of Public Health Institutes (NNPHI), OCHIN is taking part in a project using machine learning to pilot the effectiveness of new predictive analytics tools for Latent Tuberculosis Infection (LTBI) testing in a clinical setting, laying the groundwork for a variety of data-driven screening techniques in the future.
Read on to learn more about the LTBI project in our Q&A with OCHIN Epidemiologist and Senior Research Associate Jonathan V. Todd, PhD, MSPH.
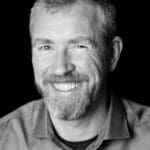
LTBI Project Q&A with OCHIN Researcher, Jonathan Todd
Q: Tell us a little about yourself. What excites you about working here at OCHIN?
A: I’m an infectious disease epidemiologist by training. I have been at OCHIN for a little over two years, and I came here because I was excited about the potential to work in a place that focuses on the safety net and patients who experience a lot of health challenges and social vulnerabilities. We’ve just started to scratch the surface on the infectious disease projects we can do here. A lot of the projects, like our Latent Tuberculosis Infection (LTBI) surveillance project, use the tools of predictive analytics.
Q: Can you share more about the LTBI project? Who did OCHIN partner with and what were you trying to accomplish?
A: We’re excited for this collaboration with the CDC, particularly because of its potential to help providers in OCHIN member clinics, and other community health centers, better manage LTBI burden with the people and communities they serve. Public health has done a pretty fantastic job, over the decades, of eliminating active tuberculosis cases in the United States, where the prevalence today is very, very low. But to eliminate TB, you must also identify those patients who are latently infected, those who don’t have symptoms. The highest prevalence of those LTBI patients is typically among patients born outside of the United States. But that’s a sensitive piece of data that isn’t routinely collected—only 11 percent of patients in the study group had recorded information on their country of birth, which alone doesn’t provide the full picture. So our first challenge was to evaluate that kind of social and demographic data, alongside the screening, diagnosis, and treatment information available in our ADVANCE Research Data Warehouse, in order to build an algorithm that would help CDC classify patients as “definite,” “probable,” and “possible” LTBI and active TB cases.
This was an incredibly complex task. We cast a very wide net, reviewing over 2 million patient records, with lots of missing data and complex medication regimens to account for, over a five-year period: 2012-2016. We are fortunate to have a really talented study team, and I am personally lucky to have the chance to work with Jon Puro, my co-PI on this project and the ADVANCE Network PI. He and the rest of the team bring a wealth of expertise analyzing complex data for public health surveillance and to inform interventions in the EHR. It is a really great team to work with.
Q: Why did CDC select OCHIN as a project partner?
A: I think CDC was interested in the size and make up of this historically underserved patient population. The ADVANCE Collaborative is well known as the nation’s largest community health center focused clinical research network, with a lot of diverse patients, as well as those who are uninsured or covered by Medicaid. CDC was looking to expand beyond its previous work in public health settings and reach patients in a different type of clinic, like Federally Qualified Health Centers, where there’s potentially a bigger burden of LTBI. So, I think the nexus between having a large data set that’s also focused on those particularly underserved populations is what triggered their interest in OCHIN.
Q: What did you learn after completing Phase 1 of this project?
A: One of our biggest findings was that there isn’t concordance, or alignment, between the data about who is screened, who is diagnosed, and who is treated. In fact, there’s really discordance in a lot of cases. So, there’s definitely an opportunity to help clinicians better recognize when to screen patients and provide follow up or treatment based on those screening results. In general, we found that OCHIN and ADVANCE have a good population for CDC to work with. There’s a high volume of patients who would be recommended for screening, based on the likely prevalence of LTBI, that are not routinely being screened; including people born outside of the U.S.; those living or working in high-risk settings, like homeless shelters or correctional facilities; and those who have HIV or for other reasons are immunosuppressed.
Q: Why is this project important for patients or beneficial to health care providers?
A: It’s all about finding that sweet spot, where you’re screening the right populations without unfairly targeting any group. By leveraging the information maintained in the EHR and developing an algorithm that’s based on that wealth of data, we can better predict the right individual candidates for LTBI screening. We can also build decision support tools for clinicians that can be seamlessly embedded into their EHR workflows. I think any time we can make the EHR interface easier for clinicians when caring for patients, by giving them a good risk predictor or screening tool to help them prioritize needs in a busy clinical setting, then that’s always better.
That’s the way a lot of our infectious disease projects are going. Step one is learning about the observational epidemiology of the disease in the patient population; step two is translating the information about the variables in our data set to predict outcomes and identify patients who might benefit from certain interventions; and step three is building tools that will help providers in our network, and other health center settings, use those interventions.
Q: Did you encounter any challenges in this project?
A: Yes, we had a lot of initial data challenges. The first one was pinpointing where in our database this particular TB screening data was housed. It wasn’t a standard variable (like age, sex, or race) that’s available in the ADVANCE Research Data Warehouse, so locating and extracting it from the back end of our EHR database was a big data challenge to begin with. A second data challenge was the high degree of “missingness,” or incomplete data. For example, with screening and diagnosis, patients might get a tuberculin skin test placed and not return to have it read. Or they might not want to reveal their country of origin, but available proxies, like preferred language, don’t necessarily reveal how long patients have lived in the United States or what their real risk of LTBI might be.
Another challenge was understanding the complexity of the TB treatment regimens, because there are a lot of different medications that can be used. Some are used only for multi-drug resistant tuberculosis, which really wasn’t a focus of this initial investigation, so there was a lot of back and forth between our analysts and the CDC team about how best to categorize them.
Carefully combining all those three different domains—screening, diagnosis, and treatment—to produce accurate risk classifications was complex and challenging, especially over a short period of time.
Q: What’s next? Any plans to scale?
A: Our next step will focus on expanding our study group to include patients through 2019, which CDC will then use to train a machine learning model that will help better predict individual patients’ risk of having LTBI, in order to guide a targeted testing approach. OCHIN researchers have also been working with our clinical informatics team to develop a suite of new clinical decision support tools, which will be piloted in the OCHIN Epic EHR. We are going to work with two clinics to test these tools in routine care, evaluate them, and help determine the best workflows. Once we’ve made sure the tools are effective and meet providers’ needs in the clinical setting, we have the technical capability to quickly make them available throughout the OCHIN network.
We can also take what we learn from this project and apply it toward screening for other more prevalent health issues. For example, OCHIN is conducting a similar study that will eventually utilize machine learning and prediction algorithms to identify patients who might benefit from pre-exposure prophylaxis (PrEP) medication to reduce their risk of HIV infection. I think there’s a lot of crossover potential for this kind of work, and this project may inform the EHR tools we build to help clinicians better manage other disease processes as well.